Themes: The State Of Generative AI - July 2023 (Pt.1)
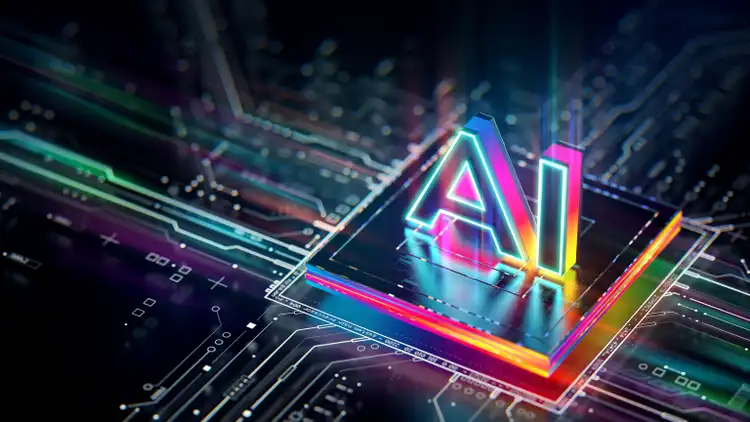
Summary
- The AI hype cycle, initiated by OpenAI's groundbreaking release of the ChatGPT model in November 2022, is not only substantial but also holds immense potential.
- Although we currently find ourselves amidst a bubble, we anticipate that several prominent enterprises will emerge, analogous to the aftermath of the Dot-com bubble.
- AI competition remains in its infancy. While the reverence for tech giants like NVIDIA Corporation (NVDA), OpenAI, and Microsoft Corporation (MSFT) is justified, they are far from invincible.
AI is running hot and has overextended to bubble territory, substantiated by the heightened VC funding landscape.
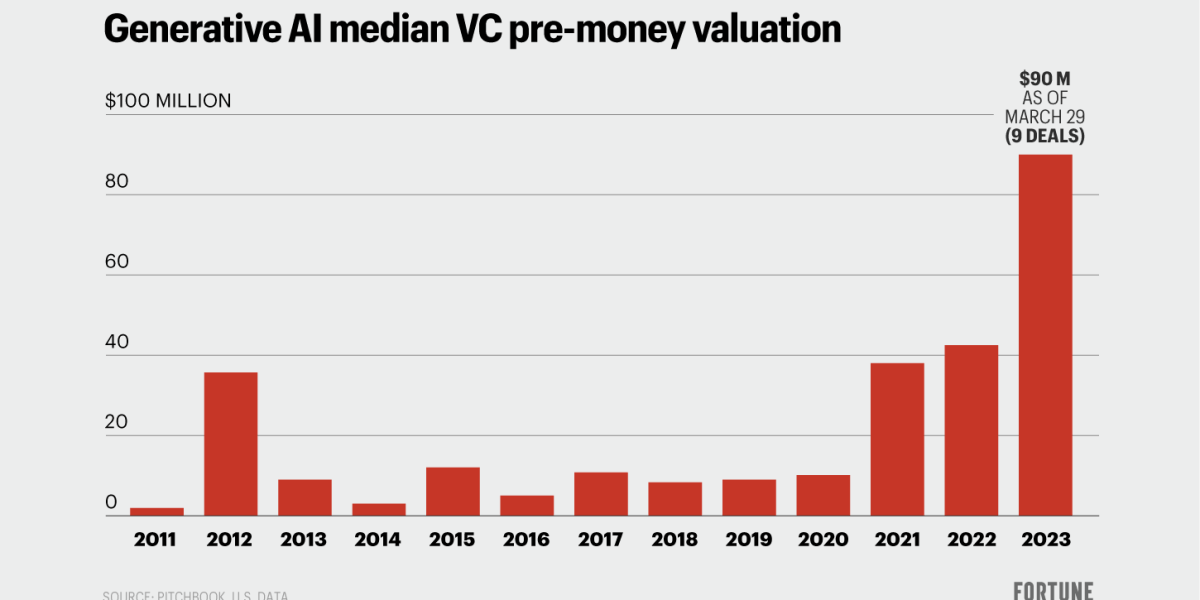
VCs are paying up as they scramble to get in on generative A.I.
Another indicator is the recent Twitter rate limiting for all users. Elon Musk is so frustrated by the amount of illegal data scraping by AI startups that he is willing to risk disturbing existing Twitter users. According to Musk, recently there has been a sharp rise in the server workload to the extent that they have to activate backup capacities. It is clear that there are too many AI startups being founded, and they are willing to break the law by crawling Twitter without consent.
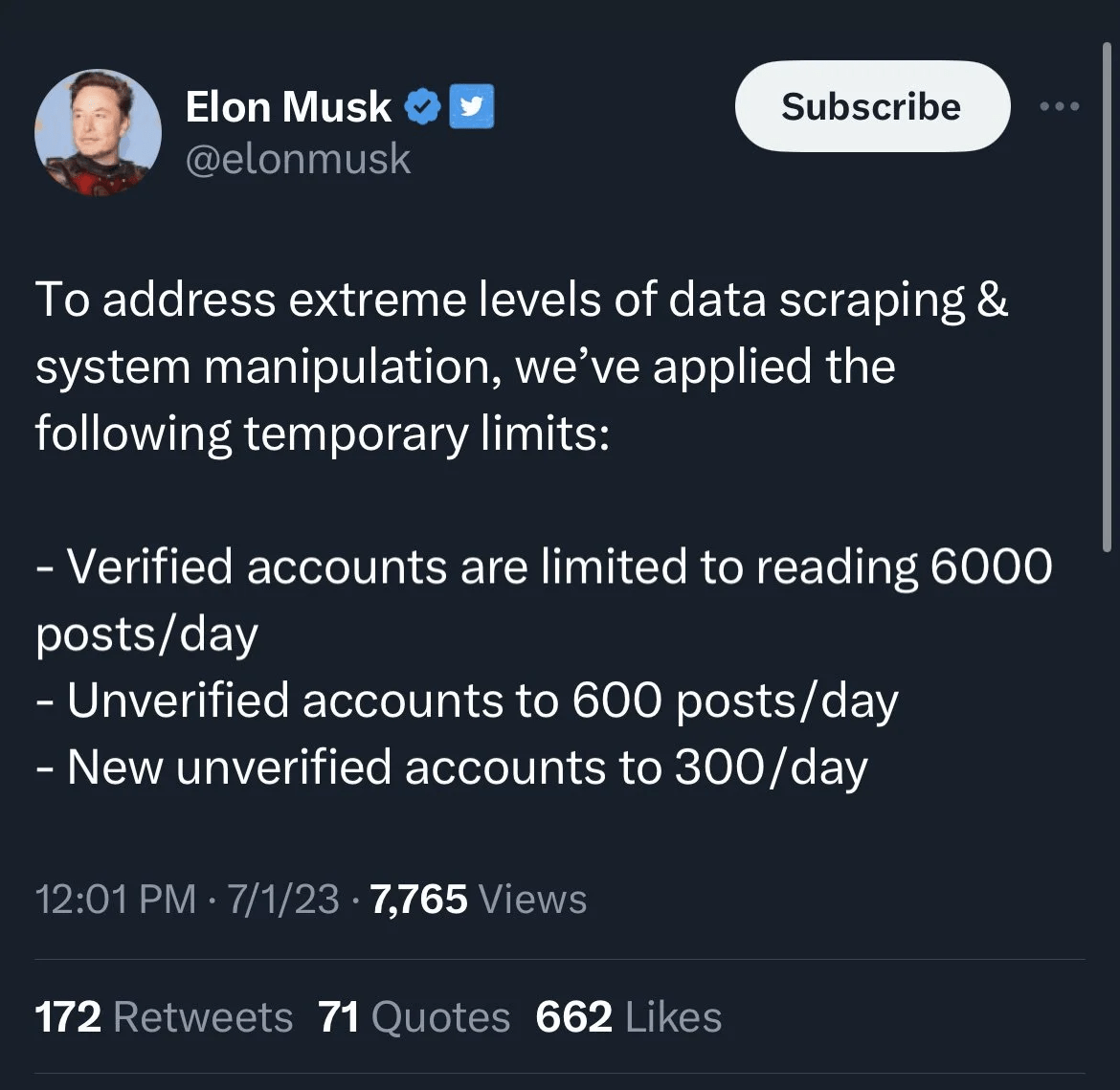
Elucidating the Concept of AI
The term 'Artificial Intelligence' often engenders confusion due to the myriad ways one can define 'intelligence.' Suppose we consider it as domain-specific intelligence, where a machine supersedes human capabilities in a particular task. In that case, AI has been in extensive production since the early 2000s. On the other hand, if we refer to it as 'Artificial General Intelligence' (AGI), a more comprehensive form of intelligence, it wasn't until the recent advent of ChatGPT that AI could replace humans in a broader range of workloads.
This ambiguity creates an ample arena for companies to manipulate marketing tactics and bewilder investors eager for quick exposure to the AI sector. However, it's important to realize that most of these firms will neither emerge as winners nor directly benefit from the propelling force of AI. Hence, understanding the nuances of AI becomes vital.
AI Segmentation
The recent surge in AI fascination isn't a novel phenomenon to us, as there has been numerous hype cycles followed by 'AI winters' since the 1980s. Yet, akin to economic development, AI is on a trajectory of growth, albeit with volatility spikes due to fluctuating expectations. For domain-specific AI, mass production has been a reality for years, with many of us unknowingly utilizing or benefiting from it in some way. These AI applications are typically developed via supervised machine learning, focusing on three critical areas:
- Computer Vision (CV) - Encompasses tasks related to image classification, recognition, editing, and autonomous driving.
- Natural Language Processing (NLP) - Deals with translation, semantic classification, sentiment analysis, and the development of chatbots.
- Recommendation Engine - Involved in search engines, content recommendations, and ranking suggestions.
AI-powered recommendation systems have been the most successful application of AI, with numerous tech behemoths experiencing significant commercial success. Examples include Google, Netflix, Facebook, Twitter, Amazon, and TikTok (operated by ByteDance). Consider TikTok's widespread go-to-market success and robust defenses, which are primarily facilitated by its underlying video recommendation engine. This engine efficiently learns user preferences, enhancing user retention by continuously improving the relevance of suggested content.
Contrary to the conventional wisdom of AI automation, recommendation algorithms are both talent and labour-intensive. They necessitate exceptional researchers capable of crafting algorithms that capture human attention and their underlying needs. Additionally, laborious manual work is required to scrape keywords and associated semantic links to enhance recommendation accuracy and relevance - a process known as 'embedded engineering.'
Similarly, NLP and CV require a significant labour investment to optimally perform. For instance, sentiment analytics requires engineers to identify key words or embeddings that suggest whether a text is leaning towards a positive or negative sentiment. Image classification, on the other hand, requires engineers to manually label images (such as distinguishing between a bird and a dog). Consequently, only a select few tech companies have the talent density and quantity to construct an ML-driven enterprise.
Understanding Machine Learning and Deep Learning
In 2012, the landscape of AI underwent a significant shift when researchers from the University of Toronto published a paper detailing a 65 million parameter deep learning model, later referred to as 'AlexNet.' Trained using two Nvidia GTX 580 gaming GPUs, this model won the ImageNet contest, where the task was to sort 1.2 million images into 1000 classes, outperforming other competitors who were still reliant on traditional Machine Learning techniques. Following this, Microsoft's 'ResNet' took the results a notch higher, outdoing human experts, thereby illustrating the potential of Deep Learning (DL) - a specialized form of Machine Learning which increases the layers, depth, and parameters within a model.
There are three foundational metrics that determine a model's performance: